How to Debug CPU Overuse in Ruby Applications
5 minute read

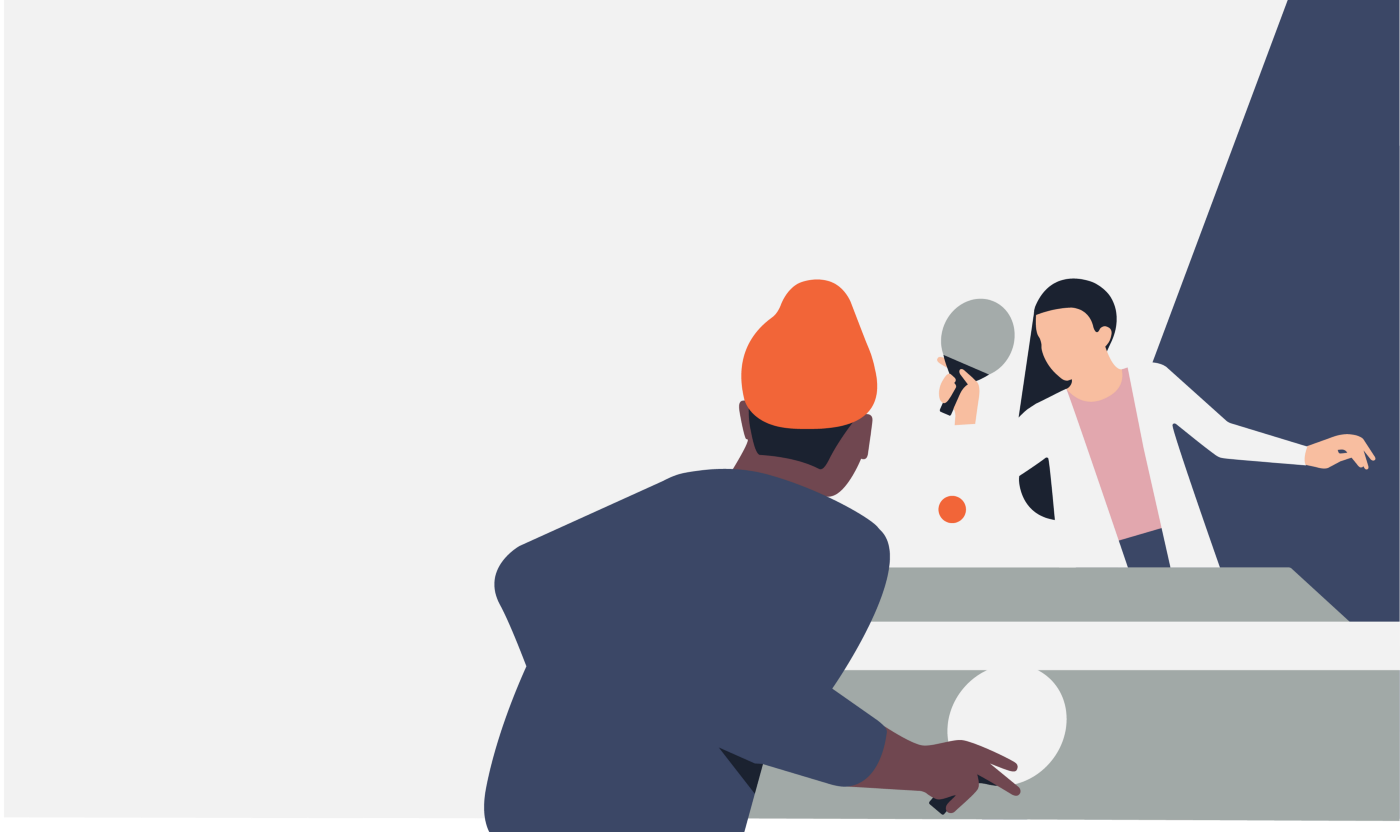
I will start by providing some context about our system, which I call System X, then describe the steps taken to discover the offending process. My hope is that you can leverage this debugging process in the future.
Context
We run a dockerized Ruby service on a Puma web server. Many of our services use Docker, Ruby, an alpine base image and MariaDB.
Before migrating to AWS, we used Prometheus for standard and custom metrics. One such metric, cpu_user_seconds_total, provided by Prometheus via CAdvisor, indicated the cumulative user CPU time consumed in seconds.
As explained here, system CPU seconds refers to the amount of CPU time used by the kernel, for tasks like interacting with hardware, memory allocation, communicating with OS processes, and managing the file system. User CPU seconds refers to time used by user space processes, like those initiated by an application or a database server, or by anything other than the kernel. For simplicity, I will refer to it as CPU utilization, as that’s what linux intends this to measure.
None of our services came close to utilizing all of it’s allotted CPU, except one…
Problem
On our production environment, the CPU utilization of the containers hosting Service X increased until it reached 100 percent. This would happen after 3 days, at which point we’d have to restart the service. The CPU utilization increased with the amount of Puma threads and HTTP requests.
Other services, some of which handle many more requests than Service X without issue, have the same dependencies with the same versions as Service X. That, coupled with the fact that user CPU was increasing, led me to believe that our application code had a problem.
Investigation process
Phase 1: Pinpoint the problematic request
Depending on the pattern of the spike, the offending process will not always be a completely synchronous, simple HTTP request. It could be a scheduled job or the processing of a message from a queue. Our pattern suggested that sustained requests to two problematic endpoints were causing the spike. The majority of requests were hitting a GET endpoint which retrieves a resource. The culprit was likely line of code hidden in that endpoint.
Phase 2: Replicate the issue on a testing environment
To verify that these endpoints were causing the spike, I ran a series of load tests on each of them, using Locust. Firing the same amount of requests to the suspect GET endpoint on our testing environment yielded the same spike! And, most importantly, the graph in CPU usage time on testing mirrored the one on production: the curve looked the same and the container also took 3 days to become unusable. To speed up the debugging process, I increased the amount of Locust requests, so that CPU usage time would spike faster:
Phase 3: Locate the offending lines of code
Now came the meat of the investigation: finding that line.
For illustration purposes, let’s say we are a note-taking app, and that the resource we are GETting will be a “notebook”. A customer can only GET his or her notebooks (not others’) and has to upgrade to Premium to be able to perform other actions, like deleting notebooks. Each notebook has an identifier, attributes and some related resources (collaborators, notes, etc).
Our application uses Grape and employs a service-repository pattern.
The request to GET /notebook/:id gets routed to a Grape class that defines a get method. That method instantiates a service class (a class responsible for the application’s business logic), passing any relevant data from the request headers. That data has been wrapped in a context object by Rack middleware earlier. It then asks the service instance to find a notebook with the id from the request parameters.
Context comes from request headers that are inserted by a different application before the request hits our notebook application. It contains the ID of the customer (so we only return a notebook belonging to that customer), the actions that customer is allowed to perform (can they get and delete notebooks? Just get them?) and the request id for logging purposes:
But as the online communication became more prevalent, it became obvious that the 127 different characters Base ASCII could display would not work for everyone. For example, the German language with its Umlaute (»Ä« or »Ö«) needed additional numbers to be defined against »Ä« and »Ö«. As a consequence, Germany and lots of other countries began to use an extended ASCII version, which used the 8th bit to unlock twice as many possible characters (254).
However, this resulted in a problem: different countries now used different extensions. The great advantage Base ASCII once had was gone. Instead of a universal character set everyone agreed upon, there were now different conflicting ASCII versions, each of them with their own special characters. Lost in translation, so to speak.
Unicode — a universal AND MORE POWERFUL SYSTEM
The solution: Unicode. Invented in the late 1980s, Unicode is a universal language that is used all over the world. Unicode’s approach follows a more complex logic but is built on Base ASCII as its first 127 characters are equal. Instead of an 8-bit system, Unicode uses a 32-bit system, allowing many more letters (up to 2 million) to be represented. Unicode is constantly updated, features signs and characters from all over the world and still has enough space to include many more new characters or emoticons.
However, the rise of Unicode came along with another major problem. The primary rule of ASCII was no longer valid. The simple logic that one byte always represented one character was abolished, as in Unicode’s 32-bit system one character could be represent by up to four bytes, creating another major translation problem.
For example, imagine a website created in the 1980s with all the text written in ASCII. On this website, every single byte represents a single character — in the format 0XXXXXXX. Now imagine that the website host wants to integrate some emoticons using Unicode. If he would simply switch to using Unicode, the entire text (written in ASCII) would be displayed incorrectly. And there is a simple reason for that error: Unicode cannot know where one character stops and another one begins as, for example, two bytes could now be only one character in Unicode instead of two in ASCII. Lost in translation. Once again.
The superhero FINALLY bridging the gap: UTF-8
For this reason, a translator was needed and, thus, UTF-8 was invented. UTF-8 is the superhero bridging the gap between ASCII and Unicode. What is so special about UTF-8 is that it is not a character set or another language, but rather a set of rules that go on top of Unicode. Simply put, UTF-8 provides a set of instructions on how to recognize where a character starts and stops and how many bytes a character has. It does so by adding some extra information at the beginning of each byte. The primary rule behind UTF-8: when a byte starts with ‘0’, it should be read as a single character. When it starts with, for example, 1110XXX, it’s the start of a sequence of three bytes representing one character.
So, if a website, originally written in ASCII, wants to add some emoticons using Unicode, it can use UTF-8 and all the content written in ASCII would still look the same, because UTF-8 ensures that all ASCII characters (0XXXXXXX) are read as one single character.
To this day, UTF-8 is still very critical for the global compatibility of technology and different systems and devices as it bridges the gap between Unicode and ASCII. At Solarisbank, our entire system is Unicode and UTF-8 compatible to ensure that the banking transactions of all our customers and partners run smoothly.
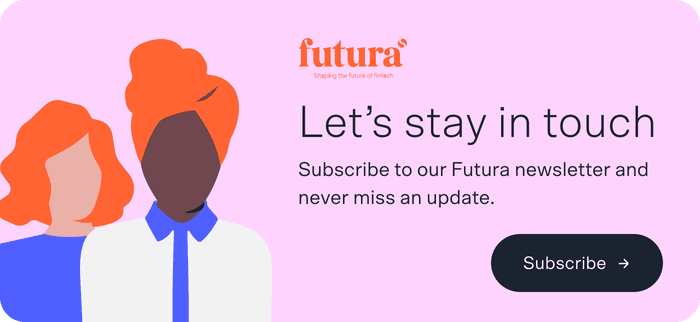